Can you use NGS panels to test for tumor mutational burden?
Written by Victoria Simms, April 24, 2023. Reviewed by Celina Whalley, April 22, 2024
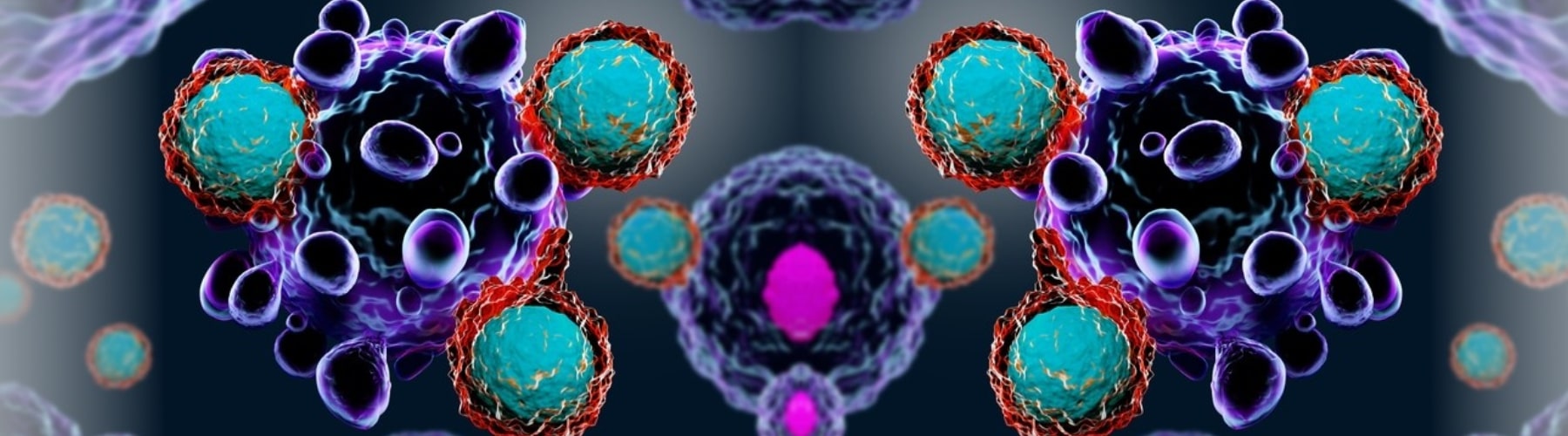
Tumor mutational burden (TMB) has been highlighted as a reliable predictor of immunotherapy response across multiple cancer types, offering the potential to help guide immunotherapy treatment decisions for cancer patients. Typically whole genome sequencing (WGS) and whole exome sequencing (WES) are used to assess TMB but next generation sequencing (NGS) panels offer a far more cost-effective method, making them a more feasible option for routine clinical use.
Biomarkers for immunotherapy treatment response
Accurately identifying biological biomarkers from within tissues of cancer patients can provide valuable insights not only into cancer cell genomics but also the tumor microenvironment and tumor-immune cell interactions. Current immune-oncology biomarkers such as programmed death-ligand 1 (PD-L1) expressed on the surface of cancer and T-cells, has shown the effectiveness of using biomarkers to identify patients who would benefit from immunotherapy treatments such as immune checkpoint inhibitors (ICIs).1
ICIs are monoclonal antibodies designed to inhibit regulatory proteins known as checkpoint proteins. By preventing PD-L1 and programmed death protein-1 (PD-1) interactions (Figure 1), an immune response can be initiated against the cancer cells.2 Although ICIs have been highly successful in increasing survival rates for subsets of cancer patients which exhibit multiple solid malignancies. Most patients treated with ICIs do not respond.
In recent years, research studies have genetically profiled cancer cells alongside evaluating tumor-immune phenotype to try and identify reliable biomarkers for ICI response. A biomarker that has been highlighted as a reliable predictor of immunotherapy response across multiple cancer types has been TMB.
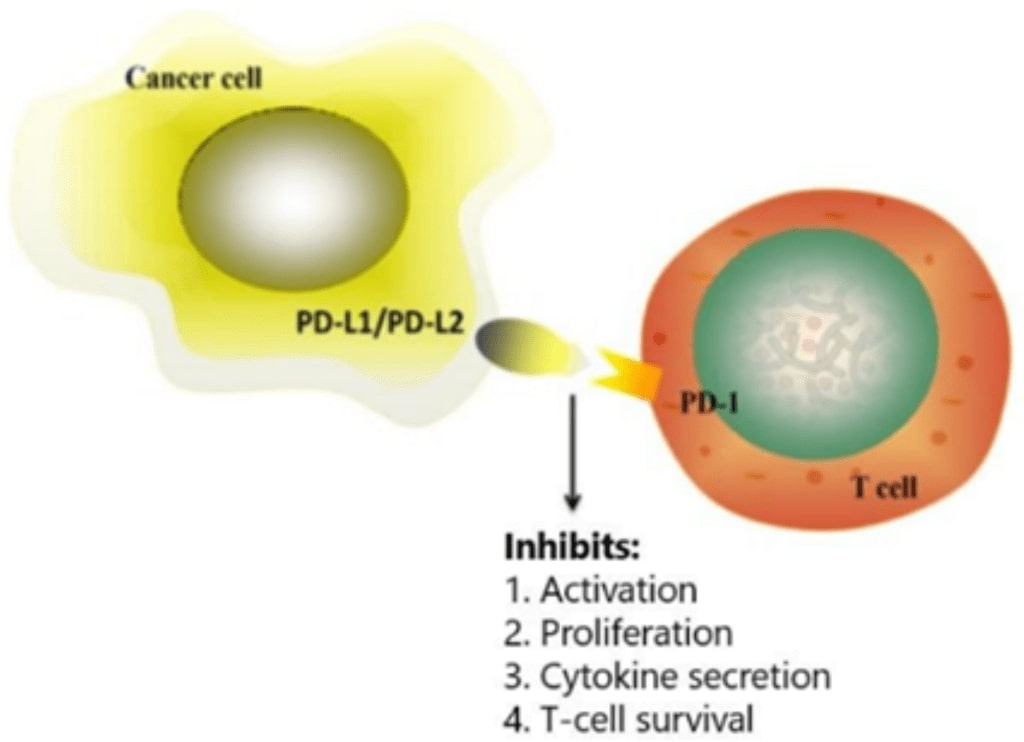
Figure 1: Programmed death-ligand 1 (PD-L1) present on the surface of cancer cells interacts with programmed cell death protein-1 (PD-1) on the surface of T-cells. Immune checkpoint inhibitors prevent the PD-L1 and PD-1 interaction allowing an immune response to be initiated against the cancer cells. Figure adapted from Han et al.2
What is tumor mutational burden (TMB)?
TMB is defined as the number of somatic mutations per megabase (Mb) of sequenced genome region and it can be assessed using NGS techniques of WGS, WES and targeted panel sequencing.
TMB is a continuous variable, often represented by a level within a tiering system of low, medium and high. A TMB score of <6 is often considered low-TMB, 6-20 is considered medium-TMB, and >20 is high-TMB. However, this tiering system is not universally defined, and studies are currently ongoing to not only define the levels within the tiering system but also to standardize methods for TMB assessment, making the comparison of TMB assessment across different NGS methods more reliable and accurate.
TMB as a biomarker for ICI response
TMB is recurrently associated with other predictive immunotherapy biomarkers such as PD-L1 expression levels and microsatellite instability (MSI). High MSI has been linked to defective DNA mismatch repair (MMR) proteins and has been widely accepted as being a reliable response predictor to ICIs. Tumors that display disregulated and defective MMRs, often exhibit high-MSI and high-TMB 3,4
High-TMB has been linked to increased expression of mutated antigens known as neoantigens, which are associated with major histocompatibility complexes (MHC) on the surface of cancer cells.5 Higher neoantigen load often correlates with enhanced T-cell responses and tumour immunogenicity. Patients with a high mutational burden, such as those with melanoma or non-small cell lung cancer (NSCLC), have reported benefits from receiving ICI treatment.6
Using targeted gene panels for TMB assessment
Comprehensive sequencing methods such as WGS and WES, have usually been considered the 'gold standard' approach for TMB analysis. These NGS techniques detect somatic mutations also known as non-synonymous mutations across the whole genome, but due to time, cost, and the resource implications of sequencing and analyzing such large datasets, these approaches are not viable for routine clinical use. Targeted gene panels provide an alternative option. For TMB analysis, a smaller more focused gene list can be used and the data extrapolated to predict the total number of somatic mutations within the coding regions across the whole genome.
So, what should be considered when selecting a NGS panel for TMB analysis?
1. Panel content
Choosing which NGS panel to select for genomic profiling can be confusing, since commercially available panels differ in size, gene content, and the mutation types that they are able to detect.
The number of genes included in off-the-shelf pan-cancer panels can vary from 300-600 genes,5 which in turn influences the target region size. At present, 375 cancer driver genes have been reported and many commercially available pan-cancer panels will try to profile as many of these genes as possible. Cancer driver genes are known to drive tumorigenesis and the identification of actionable variants is important to help guide patient treatment decisions. However, the inclusion of common cancer driver genes within the panel content and therefore the detection of driver mutations is associated with high TMB. Li et al. reported that across 19 different cancer types, the detection of driver gene mutations correlated to significantly high TMB.7
Since TMB analysis considers only somatic coding mutations, targeted gene panels focus on the detection of non-synonymous mutations, such as point mutations, small insertions and deletions (INDELs) and frameshifts. However, unlike WES, targeted panels are not limited to the inclusion of coding regions and may contain promoter and intronic regions within the panel design. These additional regions are necessary if gene fusions are to be identified, however, the specific genomic regions covered in each panel will differ and potentially influence the TMB calculation.
Alongside detecting non-synonymous mutations, the ability of the panel to detect synonymous mutations should be considered. As although synonymous mutations do not contribute to neoantigen load, their identification within the panel content can help to improve the accuracy of the TMB calculation, through reducing sampling noise, especially when paired tumor and normal samples are sequenced.8
2. Sequence region size
During the NGS panel selection process, not only should careful consideration go into the genomic regions included, but also the sequenced region size. The size of the gene panel is reported to be a critical factor in the accuracy of the TMB calculation. The panel size should be considered from a cost-benefit perspective as it is necessary to minimize any issues that could arise due to sequencing a smaller genomic region.
Evidence from several studies has suggested that for clinical samples, targeted gene panels of least 1 Mb are required for accurate TMB assessment.9-11 Calculating TMB from a NGS panel <1 Mb, results in large confidence variance, whereas larger NGS panels show significantly smaller confidence variance, giving greater accuracy in results.
"Optimizing the cost-benefit ratio, our data suggests that panels between 1.5 Mb and 3 Mb are ideally suited to estimate TMB with small confidence intervals." Buchhalter et al., 2019.
Most commercial pan-cancer panels range from 0.8-3 Mb in size. However, research suggests that only those over 1.5 Mb should be considered for accurate TMB analysis. Buchhalter et al. reports that panels of size 1.5-3 Mb are best suited for estimating TMB, due to the smaller confidence intervals.11
"The coefficient of variation of panel sequencing based estimates of TMB, decreases inversely proportional with the square root of the panel size and the square root of the TMB level." Budczies et al., 2020.
3. Pre-analytical factors and bioinformatic analysis
Several variables during the processes of library construction, NGS and bioinformatic analysis can influence panel-based TMB analysis. Several of these variables are outlined in Figure 2 and include the initial quantity and quality of the tissue and subsequent DNA sample which is inputted into the library preparation workflow, along with the enrichment technology, during the library construction process. Likewise, during sequencing, factors including genome coverage, depth of sequencing and read length may influence the accuracy of the TMB calculation.
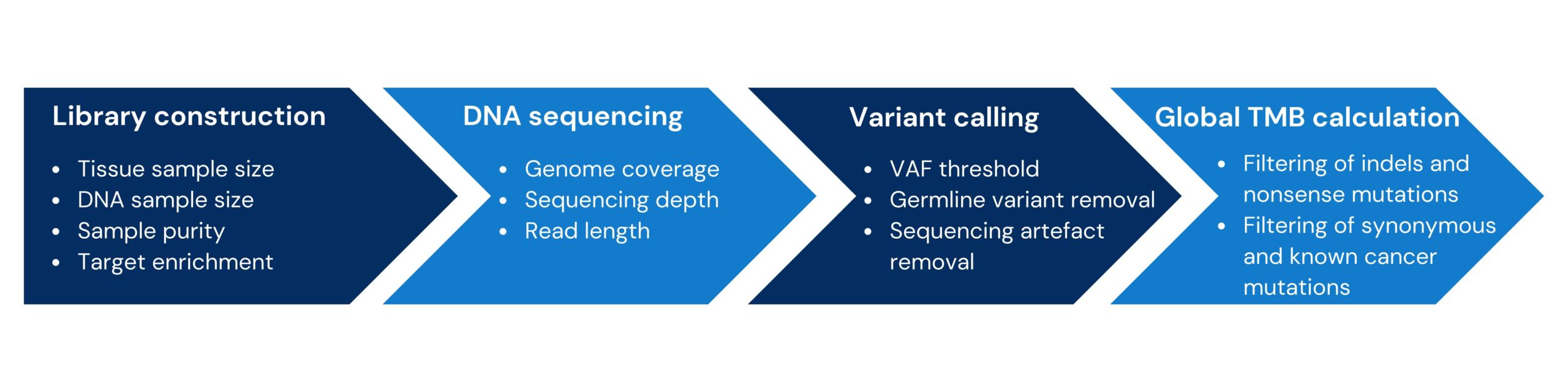
Figure 2: Overview of the factors influencing panel-based TMB quantification. Figure adapted from Fancello et al.8
Each laboratory will use their own bioinformatic pipelines and these will be optimized based on the panel specifications, for example, the sequencing method that was used and the mutation types detected. These bioinformatic algorithms will have set variable allele frequency (VAF) thresholds, along with built-in filters to remove germline variants and sequencing artefacts, to enable confident calling of somatic mutations. Along with these variables, different approaches can also be taken to extrapolate global TMB from panel-based data, the approach chosen will depend on the panel content and panel size, but it may be appropriate to filter for known cancer driver gene mutations, synonymous mutations, INDELs and nonsense mutations.
Standardizing TMB assessment
Several studies are currently ongoing to standardize TMB analysis through creating frameworks to define common TMB parameters. The latest findings from the Quality Assurance Initiative Pathology (QuIP) guidelines outline the importance of selecting a NGS panel which contains actionable mutations along with large genome coverage, ideally greater than 1 Mb. For clinical TMB reporting, and to enable TMB comparison across different NGS approaches, QuIP state that TMB should always be reported as mutations per megabase.
Genomic profiling using pan-cancer NGS panels
Comprehensive genomic profiling (CGP) using NGS panels can provide an accurate and cost-effective TMB assessment, which can help guide immunotherapy treatment decisions for cancer patients in routine clinical use.
If you are interested in performing TMB analysis using clinical samples the Nonacus GALEAS Tumor platform is a streamlined CGP workflow, from sample to data analysis.
The enhanced content of GALEAS Tumor, combined with optimized bioinformatics developed in parallel with the panel, enables scientists and clinicians to confidently detect a wide range of gene aberrations known to drive cancer, from SNVs, INDELs, selected fusions and genome-wide CNVs, to biomarkers for TMB and MSI across 519 genes.
References
- Davis AA, Patel VG. The role of PD-L1 expression as a predictive biomarker: an analysis of all US Food and Drug Administration (FDA) approvals of immune checkpoint inhibitors. Journal for Immunotherapy of Cancer. 2019;7(1):278.
- Han Y, Liu D, Li L. PD-1/PD-L1 pathway: current researches in cancer. American Journal of Cancer Research. 2020;10(3):727.
- Schrock AB, Ouyang C, Sandhu J, Sokol E, Jin D, Ross JS, et al. Tumor mutational burden is predictive of response to immune checkpoint inhibitors in MSI-high metastatic colorectal cancer. Annals of Oncology. 2019;30(7):1096-103.
- Innocenti F, Ou FS, Qu X, Zemla TJ, Niedzwiecki D, Tam R, et al. Mutational analysis of patients with colorectal cancer in CALGB/SWOG 80405 identifies new roles of microsatellite instability and tumor mutational burden for patient outcome. Journal of Clinical Oncology. 2019;37(14):1217.
- Sha D, Jin Z, Budczies J, Kluck K, Stenzinger A, Sinicrope FA. Tumor mutational burden as a predictive biomarker in solid tumors. Cancer Discovery. 2020;10(12):1808-25.
- Wang P, Chen Y, Wang C. Beyond tumor mutation burden: tumor neoantigen burden as a biomarker for immunotherapy and other types of therapy. Frontiers in Oncology. 2021;11:672677.
- Li L, Bai L, Lin H, Dong L, Zhang R, Cheng X, et al Multiomics analysis of tumor mutational burden across cancer types. Computational and Structural Biotechnology Journal. 2021;19:5637-46.
- Fancello L, Gandini S, Pelicci PG, Mazzarella L. Tumor mutational burden quantification from targeted gene panels: major advancements and challenges. Journal for Immunotherapy of Cancer. 2019;1-3.
- Chalmers ZR, Connelly CF, Fabrizio D, Gay L, Ali SM, Ennis R, et al. Analysis of 100,000 human cancer genomes reveals the landscape of tumor mutational burden. Genome Medicine. 2017;1-4.
- Garofalo A, Sholl L, Reardon B, Taylor-Weiner A, Amin-Mansour A, Miao D, et al. The impact of tumor profiling approaches and genomic data strategies for cancer precision medicine. Genome Medicine. 2016;1-0.
- Buchhalter I, Rempel E, Endris V, Allgäuer M, Neumann O, Volckmar AL, et al. Size matters: dissecting key parameters for panel‐based tumor mutational burden analysis. International Journal of Cancer. 2019;144(4):848-58.
- Budczies J, Allgäuer M, Litchfield K, Rempel E, Christopoulos P, Kazdal D, et al. Optimizing panel-based tumor mutational burden (TMB) measurement. Annals of Oncology.2019;30(9):1496-506.
- Budczies J, Kazdal D, Allgäuer M, Christopoulos P, Rempel E, Pfarr N, et al. Quantifying potential confounders of panel-based tumor mutational burden (TMB) measurement. Lung Cancer. 2020;142:114-9.